Business
Understanding Data Quality in Terms of Errors and Ways to Tackle it
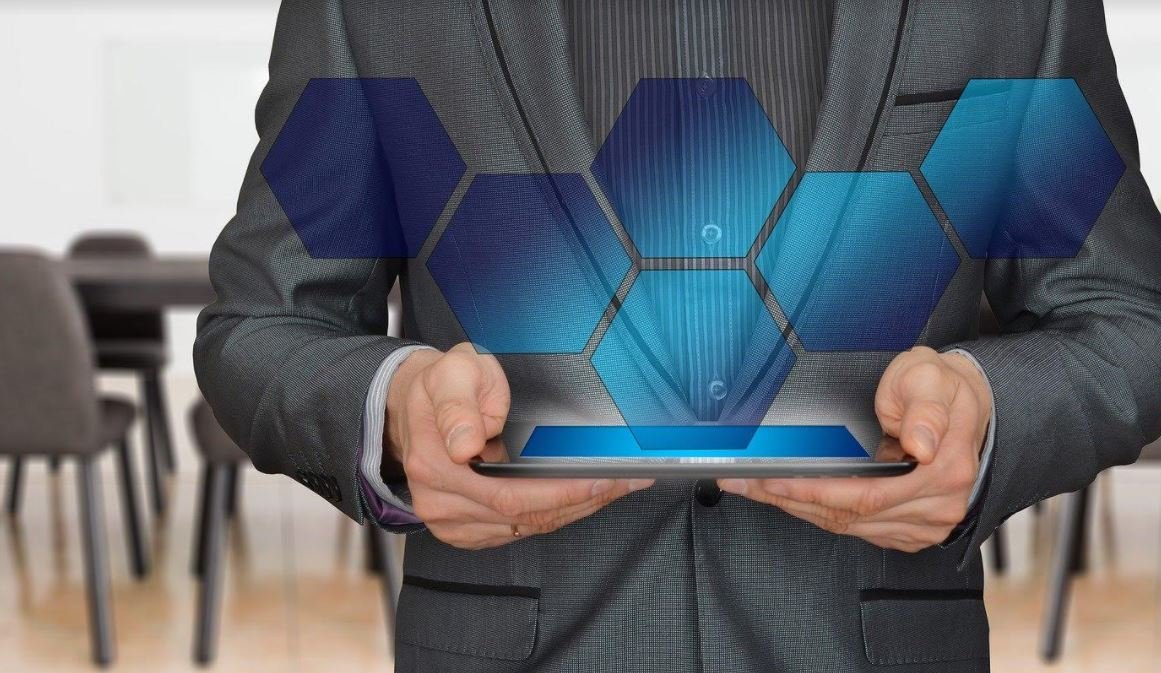
Data quality is important for all types of businesses, and it is critical in terms of enterprise database management. As you know, one has to understand what it will take to ensure the quality of data, and we should also be aware of the issues related to data quality.
As we can briefly define, data quality is the ability to give quality data sets to serve whichever needs the company intends to use the data for. This may be sending the marketing materials to the customers, better managing the prospective leads to cover those into sales or to manage the customer data for better support services, etc.
No matter what your data use case is, data quality is very important. Without quality being assured, the given data cannot fulfill the purpose. For example, if there are errors in the database with addresses, you cannot effectively reach the customers. A database with phone numbers that do not include area codes may fall short of offering any valuable information to use in real-time.
[lwptoc]
Common causes of data quality problems
Now, as you outline what data quality is and can visualize what it looks like in real-time applications, let us go deeper into various types of problems that may lead to shortcomings in terms of data quality. Further, we will discuss six common ways through which data quality errors may creep into an organization’s data operations, even if you adhere to the best practices in managing and analyzing data.
-
Errors in manual entry
Humans tend to make mistakes, and even a smaller data set, which includes the data entered manually by humans, is likely to contain the data entered manually by the humans and likely to contain the mistakes. There can be typos and entry of wrong data into the fields and also missed entries which are inevitable in manual data entry.
-
Optical Character Recognition related errors
Machines also tend to make mistakes while doing data entry. In this case, organizations must try to digitize larger amounts of data quickly. For this, they often rely on OCR or Optical Character Recognition. This is a technology used to scan images and extracts data text from those. This can be very useful if you want to evaluate thousands of answer sheets or take addresses marked in an entry form. All these data can be entered into a digital database so that you can easily analyze those using Hadoop.
However, OCR is also not fully perfect. If there are thousands of text lines to scan, you may have certain errors in terms of words or characters being misinterpreted. For example, it is possible that zero is being read as eight, or some proper nouns are misinterpreted as common words, etc. These types of issues can also arise with another type of automated data entry like text-to-speech or so. For adopting data quality tools and database error handling, you can take the consultation of RemoteDBA.
-
Incomplete information
While compiling various data sets, there may also be problems like not having information for all entries. For example, there can be a database of addresses, which may not have a zip code for some addresses, which also cannot be determined through any automation methods used to compile the dataset.
-
Ambiguous data
While building up a database, you may also find that some of your data is ambiguous and leads to uncertainty about how and where to enter it. For example, if the database is meant to enter phone numbers, then some of the numbers you try to enter may not be standard ten digital numbers. It is difficult to determine whether the extra digital are simply typos or any other international numbers which have more than ten digitals. In that case, need to also check whether that number contains international dialing information too? There are various such ambiguity issues to be addressed. All such challenges are hard to answer systematically and instantly when you are working with a huge volume of data.
-
Data duplication
Data duplication is a very common scenario in enterprise data management. You may end up finding there are two or more similar entries in the data tables. For example, you may find two entries of the same name living in the same address. It becomes difficult to understand whether this is duplicate info or maybe like two people with the same name (as a father and son) living at the same address. You have to sort the seemingly duplicate data in order to get the best output from the available data.
-
Errors in data transformation
Converting data from one format to another may lead to some mistakes. We can consider an example of converting spreadsheet data into comma-separated value or CSV file. As the data fields inside the CSV files are separate using a comma, you may sometimes run into problems while performing the conversion in such an event that some of the data entries in the spreadsheet may already contain a comma in it.
Unless you have very strong data conversion tools, it is not easy to know the small differences like a comma, which is supposed to distinguish between two data fields. These things also may get more complicated when you perform more complex data conversions like taking the mainframe database, which has become so popular over the last few years.
-
Correcting the data quality errors
These are distinct types of data quality mistakes that are so difficult to downplay. In fact, the best approach to think about data quality-related issues is by recognizing them as inevitable and the need to handle them well. The best way to handle data quality issues is by taking it as a part of the database management practices and tackling it through the best possible measures.
These are not usually because of the database management process laws, but the problems arise from within the data itself. Fortunately, there are many solutions to tackle these. You have to introduce precise data integration practices and advanced data quality tools, which will help minimize the number of errors introduced during data conversion processes. With such tools, you can find these issues at the first point and also fix the data quality challenges instantly.
Read more: Drive Your Business with Innovative Product Design
-
Celebrity4 weeks ago
Is YNW Melly Out Of Jail? What Is The YNW Melly Release Date, Career, Early Life, And More
-
Sports4 weeks ago
More Than Just a Game: How College Sports Can Shape Your Future
-
Tech3 weeks ago
AI Software: Transforming the Future of Technology
-
Tech3 weeks ago
All About Com. Dti. Folder Launcher: Features, Benefits, Tips, And More